Predictive analytics for hiring uses modern data and assessment science to project candidates’ fit and likelihood for success based on positive behavioural patterns among current employees
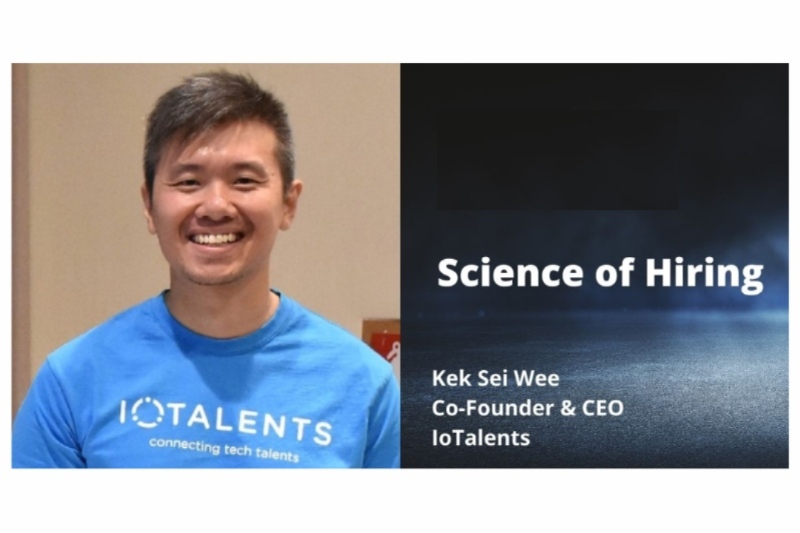
Ask any HR recruitment professional and they will tell you that hiring predictors are difficult to get right. In fact, the majority of today’s organizations struggle to accurately identify the right person for the job. If it is already expensive to hire, the cost of bad hire is even higher. Today, 95% of organizations admit to making bad hires every year, according to a research by Brandon Hall Group.
An analysis published in Psychological Bulletin concluded that statistically, a typical employment interview leads to the correct hire only 57% of the time. Despite so much being done to assess and select the candidate, the odds of a right hire are only slightly better than the flipping of a coin. But employers must still make hiring decisions.
Even when there are no hiring predictors that can guarantee 100% success, some are definitely worth looking at. Examples of better hiring predictors includes critical thinking and cognitive abilities, skills, conscientiousness (one of the five personality traits of the Big Five personality theory) and past behavior (real world examples to support their claims).
On the other hand, some of the worst predictors cited include first impressions and school grades. To make sense out of the importance of job fit or performance of hires, it is often not about the lack of skills or ability, but rather lack of “fit with job” itself, the hiring manager or the teams the person has been assigned to (as observed from our experience with our recruitment clients).
So, what can a hirer do to improve the odds and build a more effective and reliable hiring process. How can technology and data help?
Google was one of the first companies to effectively utilize predictive analytics in its hiring process, collecting all the possible measurable data and test scores they could find. However, when they analysed actual job performance, they found that school grades are one of the worst predictors for hiring (according to Laszlo Bock, former SVP of People Operations). The tech giant has invested significant resources in developing analytical models that support human decision-making. In particular, one of the team’s most interesting findings is that the company experienced little benefit to conducting more than four rounds of interviews. Instead, they today make use of a hiring committee where hiring decisions are made through a team consensus.
Predictive analytics for hiring uses modern data and assessment science to project candidates’ fit and likelihood for success based on positive behavioural patterns among current employees. Instead of traditional hiring, which relies on interviews based mainly on intuition and gut feel, predictive hiring relies on data points and smart algorithms to recommend best fit candidates to recruiters and hiring managers.
But the truth is, it will always be difficult to predict fit and performance, because humans are complex and humans interacting in human systems are even more complex.
Hiring is hard, expensive, time-consuming, and inherently uncertain. And nobody is very good at doing it alone, whether you are a Google manager, a start-up founder, or a sports manager. When it comes to identifying the best talent, groups’ decisions are better than individuals’, and having data is always better than not having it at all.
Apart from identifying the best talent, it is equally important to have means to access to the talent pool (both active and passive). Researchers have long shown that referrals can surface better job candidates. Referred candidates are more likely to get call backs, more likely to be hired, and more likely to stay at the company. Hiring is like attending a blind date, and referral will be the introduction. They give both sides a little bit more certainty and information about fit. A study on the value of hiring through referral concludes that referrals work because they yield better fits, not because they yield smarter workers. Today referrals now account for 30-50% of all hires at large companies, according to a recent paper. Most referrals also come pre-vetted and cost less to find.
The biggest disruptor in recruitment today is experimentation with HRTech solutions and services. Recruiting has also become a digital experience as candidates expect convenience and mobile contact.
At IoTalents, we develop HR Tech ideas and solutions centred around cognitive technologies such as artificial intelligence (AI), process automation, natural language processing, and predictive algorithms. We create objective, data-driven profiling tools, allowing clients to target and surface high-fit candidates. We also optimise sourcing channels through our popular and effective referral-based recruitment platform, Jobs007, to get better referrals for better recruitment outcomes.
About the Author:

Sei Wee is the founder and CEO of IoTalents. As IoTalents’ chief driving force, he leads the company in its goals to become an innovator and game-changer in the HR recruitment sphere, all through the empowering use of technology. As a serial entrepreneur, Sei Wee has a proven track record of success when it comes to business launches, and has at his disposal a network of invaluable resources built up over the years. This is a estament to his high-energy performance-driven leadership and strong work ethics. He sees IoTalents making a difference through HR Tech, and is also immensely passionate on the trend that an exploding shared economy of global IT talents will reshape the workforce of the future.